Is there any special case where ridge regression can shrink coefficients to zero?
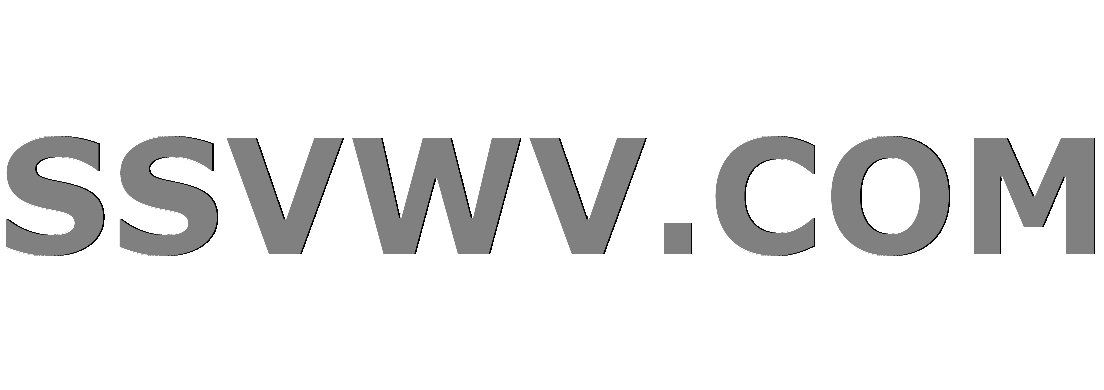
Multi tool use
Is there any special case where ridge regression can shrink coefficients to zero?
Are there some special cases, where the Ridge Regression can also lead to coefficients that are zero ?
It is widely known, that lasso is shrinking coefficients towards or on zero, while the ridge Regression cant shrink coefficients to zero
In which cases an ols coefficient can be exactly zero ?
– Vala
Aug 28 at 18:54
This will happen whenever the response variable is orthogonal to each of the explanatory variables.
– whuber♦
Aug 28 at 18:56
Would there be also the requirement that the predictors are orthogonal to each other, or would it be enough if just the correlation to the respone is zero
– Vala
Aug 28 at 18:57
See also stats.stackexchange.com/questions/74542/… for an explanation of why Ridge cannot shrink the parameters to zero (unless they start there, as @whuber observes.)
– jbowman
Aug 28 at 20:14
1 Answer
1
Suppose, as in the case of least squares methods, you are trying to solve a statistical estimation problem for a (vector-valued) parameter $beta$ by minimizing an objective function $Q(beta)$ (such as the sum of squares of the residuals). Ridge Regression "regularizes" the problem by adding a non-negative linear combination of the squares of the parameter, $P(beta).$ $P$ is (obviously) differentiable with a unique global minimum at $beta=0.$
The question asks, when is it possible for the global minimum of $Q+P$ to occur at $beta=0$? Assume, as in least squares methods, that $Q$ is differentiable in a neighborhood of $0.$ Because $0$ is a global minimum for $Q+P$ it is a local minimum, implying all its partial derivatives are $0.$ The sum rule of differentiation implies
$$fracpartialpartial beta_i(Q(beta) + P(beta)) = fracpartialpartial beta_iQ(beta) + fracpartialpartial beta_iP(beta) = Q_i(beta) + P_i(beta)$$
is zero at $beta=0.$ But since $P_i(0)=0$ for all $i,$ this implies $Q_i(0)=0$ for all $i,$ which makes $0$ at least a local minimum for the original objective function $Q.$ In the case of any least squares technique every local minimum is also a global minimum. This compels us to conclude that
Quadratic regularization of Least Squares procedures ("Ridge Regression") has $beta=0$ as a solution if and only if $beta=0$ is a solution of the original unregularized problem.
As pointed out by Martijn Weterings, it would also shrink coefficients to zero if t=0, or lambda converges to infinity. Regarding to the latter one: Could Ridge shrink a coefficient to zero for a sufficient large Tuning Parameter or is it just a theoretical concept that if lambda converges to infinity then the coefficient will be converge also to zero
– Vala
Aug 28 at 19:14
Lambda going to infinity is the equivalent of minimizing $Q/lambda + P.$ I hope it's easy to see that for sufficiently large $lambda$ the solutions will have to be close to $beta=0,$ guaranteeing convergence to $beta=0$ in the limit.
– whuber♦
Aug 28 at 19:20
There will be also close to zero, but cant get exactly zero, Right ?
– Vala
Aug 28 at 19:34
Please re-read the conclusion of my answer: I can't think of any way to make it clearer.
– whuber♦
Aug 28 at 19:36
Regarding to your final conclusion it should be correct
– Vala
Aug 28 at 19:39
By clicking "Post Your Answer", you acknowledge that you have read our updated terms of service, privacy policy and cookie policy, and that your continued use of the website is subject to these policies.
Of course! If the least squares estimates are zero, then Ridge Regression will always produce zeros. What would be of interest is to find any other situation :-).
– whuber♦
Aug 28 at 18:53