Tensorflow: copy existing graph into new graph multiple times
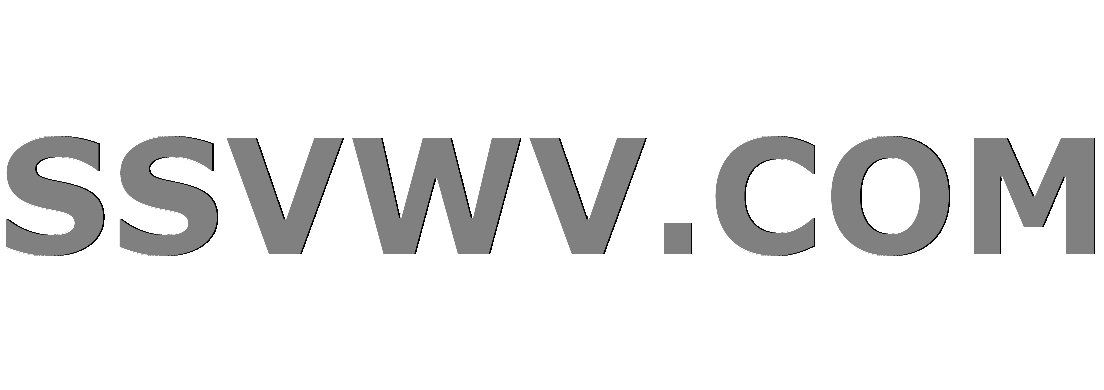
Multi tool use
up vote
1
down vote
favorite
I want to paste an existing tensorflow graph into a new graph.
Suppose I create a graph computing y = tanh(x @ w)
import tensorflow as tf
import numpy as np
def some_function(x):
w = tf.Variable(initial_value=np.random.randn(4, 5), dtype=tf.float32)
return tf.tanh(x @ w)
x = tf.placeholder(shape=(None, 4), dtype = tf.float32)
y = some_function(x)
sess = tf.Session()
sess.run(tf.global_variables_initializer())
val_x = np.random.randn(3, 4)
val_y, = sess.run([y], feed_dict=x: val_x)
Great. Now suppose I've lost the code that generated that graph, but I still have access to variables (x
, y
). Now I want to take this graph (using the current value of w), and copy it twice into a new graph (the two paths should share the same w
), so that I now compute d = tf.reduce_sum((tanh(x1 @ w)-tanh(x2 @ w))**2)
by adding the line:
# Starting with access to tensors: x, y
<SOMETHING HERE>
d = tf.reduce_sum((y1-y2)**2)
val_x1 = np.random.randn(3, 4)
val_x2 = np.random.randn(3, 4)
val_d = sess.run([d], feed_dict = x1: val_x1, x2: val_x2)
What do I fill in for <SOMETHING HERE>
to make this work? (Obviously, without recreating the first graph)
python tensorflow
add a comment |
up vote
1
down vote
favorite
I want to paste an existing tensorflow graph into a new graph.
Suppose I create a graph computing y = tanh(x @ w)
import tensorflow as tf
import numpy as np
def some_function(x):
w = tf.Variable(initial_value=np.random.randn(4, 5), dtype=tf.float32)
return tf.tanh(x @ w)
x = tf.placeholder(shape=(None, 4), dtype = tf.float32)
y = some_function(x)
sess = tf.Session()
sess.run(tf.global_variables_initializer())
val_x = np.random.randn(3, 4)
val_y, = sess.run([y], feed_dict=x: val_x)
Great. Now suppose I've lost the code that generated that graph, but I still have access to variables (x
, y
). Now I want to take this graph (using the current value of w), and copy it twice into a new graph (the two paths should share the same w
), so that I now compute d = tf.reduce_sum((tanh(x1 @ w)-tanh(x2 @ w))**2)
by adding the line:
# Starting with access to tensors: x, y
<SOMETHING HERE>
d = tf.reduce_sum((y1-y2)**2)
val_x1 = np.random.randn(3, 4)
val_x2 = np.random.randn(3, 4)
val_d = sess.run([d], feed_dict = x1: val_x1, x2: val_x2)
What do I fill in for <SOMETHING HERE>
to make this work? (Obviously, without recreating the first graph)
python tensorflow
What is it that you start with exactly? AGraphDef
? Or just some graph in memory from where you want to duplicate a subgraph (delimited by an input and an output) and connect it somewhere else? Also do you need to do this while you are creating the graph or within an alive session?
– jdehesa
Nov 8 at 14:23
I start with access to tensorsx
,y
, and nothing else (edited question to clarify, thanks)
– Peter
Nov 8 at 14:25
add a comment |
up vote
1
down vote
favorite
up vote
1
down vote
favorite
I want to paste an existing tensorflow graph into a new graph.
Suppose I create a graph computing y = tanh(x @ w)
import tensorflow as tf
import numpy as np
def some_function(x):
w = tf.Variable(initial_value=np.random.randn(4, 5), dtype=tf.float32)
return tf.tanh(x @ w)
x = tf.placeholder(shape=(None, 4), dtype = tf.float32)
y = some_function(x)
sess = tf.Session()
sess.run(tf.global_variables_initializer())
val_x = np.random.randn(3, 4)
val_y, = sess.run([y], feed_dict=x: val_x)
Great. Now suppose I've lost the code that generated that graph, but I still have access to variables (x
, y
). Now I want to take this graph (using the current value of w), and copy it twice into a new graph (the two paths should share the same w
), so that I now compute d = tf.reduce_sum((tanh(x1 @ w)-tanh(x2 @ w))**2)
by adding the line:
# Starting with access to tensors: x, y
<SOMETHING HERE>
d = tf.reduce_sum((y1-y2)**2)
val_x1 = np.random.randn(3, 4)
val_x2 = np.random.randn(3, 4)
val_d = sess.run([d], feed_dict = x1: val_x1, x2: val_x2)
What do I fill in for <SOMETHING HERE>
to make this work? (Obviously, without recreating the first graph)
python tensorflow
I want to paste an existing tensorflow graph into a new graph.
Suppose I create a graph computing y = tanh(x @ w)
import tensorflow as tf
import numpy as np
def some_function(x):
w = tf.Variable(initial_value=np.random.randn(4, 5), dtype=tf.float32)
return tf.tanh(x @ w)
x = tf.placeholder(shape=(None, 4), dtype = tf.float32)
y = some_function(x)
sess = tf.Session()
sess.run(tf.global_variables_initializer())
val_x = np.random.randn(3, 4)
val_y, = sess.run([y], feed_dict=x: val_x)
Great. Now suppose I've lost the code that generated that graph, but I still have access to variables (x
, y
). Now I want to take this graph (using the current value of w), and copy it twice into a new graph (the two paths should share the same w
), so that I now compute d = tf.reduce_sum((tanh(x1 @ w)-tanh(x2 @ w))**2)
by adding the line:
# Starting with access to tensors: x, y
<SOMETHING HERE>
d = tf.reduce_sum((y1-y2)**2)
val_x1 = np.random.randn(3, 4)
val_x2 = np.random.randn(3, 4)
val_d = sess.run([d], feed_dict = x1: val_x1, x2: val_x2)
What do I fill in for <SOMETHING HERE>
to make this work? (Obviously, without recreating the first graph)
python tensorflow
python tensorflow
edited Nov 8 at 14:25
asked Nov 8 at 14:11


Peter
3,46842740
3,46842740
What is it that you start with exactly? AGraphDef
? Or just some graph in memory from where you want to duplicate a subgraph (delimited by an input and an output) and connect it somewhere else? Also do you need to do this while you are creating the graph or within an alive session?
– jdehesa
Nov 8 at 14:23
I start with access to tensorsx
,y
, and nothing else (edited question to clarify, thanks)
– Peter
Nov 8 at 14:25
add a comment |
What is it that you start with exactly? AGraphDef
? Or just some graph in memory from where you want to duplicate a subgraph (delimited by an input and an output) and connect it somewhere else? Also do you need to do this while you are creating the graph or within an alive session?
– jdehesa
Nov 8 at 14:23
I start with access to tensorsx
,y
, and nothing else (edited question to clarify, thanks)
– Peter
Nov 8 at 14:25
What is it that you start with exactly? A
GraphDef
? Or just some graph in memory from where you want to duplicate a subgraph (delimited by an input and an output) and connect it somewhere else? Also do you need to do this while you are creating the graph or within an alive session?– jdehesa
Nov 8 at 14:23
What is it that you start with exactly? A
GraphDef
? Or just some graph in memory from where you want to duplicate a subgraph (delimited by an input and an output) and connect it somewhere else? Also do you need to do this while you are creating the graph or within an alive session?– jdehesa
Nov 8 at 14:23
I start with access to tensors
x
, y
, and nothing else (edited question to clarify, thanks)– Peter
Nov 8 at 14:25
I start with access to tensors
x
, y
, and nothing else (edited question to clarify, thanks)– Peter
Nov 8 at 14:25
add a comment |
1 Answer
1
active
oldest
votes
up vote
1
down vote
accepted
There is the Graph Editor module to help with this sort of operations. Its main disadvantage is that you cannot have a running session while you modify the graph. However, you can checkpoint the session, modify the graph and the restore it back if you need so.
The problem with what you want is that you basically need to replicate a subgraph except you do no want to replicate variables. So you can simply exclude variable types (mainly Variable
, VariableV2
and maybe VarHandleOp
, although I threw in a few more I found in TensorFlow code). You can do that with a function like this:
import tensorflow as tf
# Receives the outputs to recalculate and the input replacements
def replicate_subgraph(outputs, mappings):
# Types of operation that should not be replicated
# Taken from tensorflow/python/training/device_setter.py
NON_REPLICABLE = 'Variable', 'VariableV2', 'AutoReloadVariable',
'MutableHashTable', 'MutableHashTableV2',
'MutableHashTableOfTensors', 'MutableHashTableOfTensorsV2',
'MutableDenseHashTable', 'MutableDenseHashTableV2',
'VarHandleOp', 'BoostedTreesEnsembleResourceHandleOp'
# Find subgraph ops
ops = tf.contrib.graph_editor.get_backward_walk_ops(outputs, stop_at_ts=mappings.keys())
# Exclude non-replicable operations
ops_replicate = [op for op in ops if op.type not in NON_REPLICABLE]
# Make subgraph viewitems
sgv = tf.contrib.graph_editor.make_view(*ops_replicate)
# Make the copy
_, info = tf.contrib.graph_editor.copy_with_input_replacements(sgv, mappings)
# Return new outputs
return info.transformed(outputs)
For an example similar to yours (I edited it a bit so it is easy to see that the output is correct because the second value is ten times the first one).
import tensorflow as tf
def some_function(x):
w = tf.Variable(initial_value=tf.random_normal((5,)), dtype=tf.float32)
return 2 * (x * w)
x1 = tf.placeholder(shape=(), dtype=tf.float32, name='X1')
x2 = tf.placeholder(shape=(), dtype=tf.float32, name='X2')
y1 = some_function(x1)
y2, = replicate_subgraph([y1], x1: x2)
init = tf.global_variables_initializer()
with tf.Session() as sess:
sess.run(init)
print(*sess.run([y1, y2], feed_dict=x1: 1, x2: 10), sep='n')
Output:
[ 2.3356955 2.277849 0.58513653 2.0919807 -0.15102367]
[23.356955 22.77849 5.851365 20.919807 -1.5102367]
EDIT:
Here is another solution using tf.make_template
. This requires you to actually have the code for the function, but it is a cleaner and "more official" way of supporting subgraph reuse.
import tensorflow as tf
def some_function(x):
w = tf.get_variable('W', (5,), initializer=tf.random_normal_initializer())
# Or if the variable is only local and not trainable
# w = tf.Variable(initial_value=tf.random_normal(5,), dtype=tf.float32, trainable=False)
return 2 * (x * w)
x1 = tf.placeholder(shape=(), dtype=tf.float32, name='X1')
x2 = tf.placeholder(shape=(), dtype=tf.float32, name='X2')
some_function_tpl = tf.make_template('some_function', some_function)
y1 = some_function_tpl(x1)
y2 = some_function_tpl(x2)
init = tf.global_variables_initializer()
with tf.Session() as sess:
sess.run(init)
print(*sess.run([y1, y2], feed_dict=x1: 1, x2: 10), sep='n')
Nice! Thanks a lot. Now the only thing that's unclear is how you do this when the "subgraph" already has values associated with the variables (eg. you restored the state withnew_saver = tf.train.import_meta_graph(...)
andnew_saver.restore(sess, ...)
because it seems you can't do this with an active session..
– Peter
Nov 8 at 15:38
@Peter Yes, with Graph Editor you would have to save and close the session before editing and restore it back after. But the variable object is really the same, so everything should work fine if you do that.
– jdehesa
Nov 8 at 15:54
add a comment |
1 Answer
1
active
oldest
votes
1 Answer
1
active
oldest
votes
active
oldest
votes
active
oldest
votes
up vote
1
down vote
accepted
There is the Graph Editor module to help with this sort of operations. Its main disadvantage is that you cannot have a running session while you modify the graph. However, you can checkpoint the session, modify the graph and the restore it back if you need so.
The problem with what you want is that you basically need to replicate a subgraph except you do no want to replicate variables. So you can simply exclude variable types (mainly Variable
, VariableV2
and maybe VarHandleOp
, although I threw in a few more I found in TensorFlow code). You can do that with a function like this:
import tensorflow as tf
# Receives the outputs to recalculate and the input replacements
def replicate_subgraph(outputs, mappings):
# Types of operation that should not be replicated
# Taken from tensorflow/python/training/device_setter.py
NON_REPLICABLE = 'Variable', 'VariableV2', 'AutoReloadVariable',
'MutableHashTable', 'MutableHashTableV2',
'MutableHashTableOfTensors', 'MutableHashTableOfTensorsV2',
'MutableDenseHashTable', 'MutableDenseHashTableV2',
'VarHandleOp', 'BoostedTreesEnsembleResourceHandleOp'
# Find subgraph ops
ops = tf.contrib.graph_editor.get_backward_walk_ops(outputs, stop_at_ts=mappings.keys())
# Exclude non-replicable operations
ops_replicate = [op for op in ops if op.type not in NON_REPLICABLE]
# Make subgraph viewitems
sgv = tf.contrib.graph_editor.make_view(*ops_replicate)
# Make the copy
_, info = tf.contrib.graph_editor.copy_with_input_replacements(sgv, mappings)
# Return new outputs
return info.transformed(outputs)
For an example similar to yours (I edited it a bit so it is easy to see that the output is correct because the second value is ten times the first one).
import tensorflow as tf
def some_function(x):
w = tf.Variable(initial_value=tf.random_normal((5,)), dtype=tf.float32)
return 2 * (x * w)
x1 = tf.placeholder(shape=(), dtype=tf.float32, name='X1')
x2 = tf.placeholder(shape=(), dtype=tf.float32, name='X2')
y1 = some_function(x1)
y2, = replicate_subgraph([y1], x1: x2)
init = tf.global_variables_initializer()
with tf.Session() as sess:
sess.run(init)
print(*sess.run([y1, y2], feed_dict=x1: 1, x2: 10), sep='n')
Output:
[ 2.3356955 2.277849 0.58513653 2.0919807 -0.15102367]
[23.356955 22.77849 5.851365 20.919807 -1.5102367]
EDIT:
Here is another solution using tf.make_template
. This requires you to actually have the code for the function, but it is a cleaner and "more official" way of supporting subgraph reuse.
import tensorflow as tf
def some_function(x):
w = tf.get_variable('W', (5,), initializer=tf.random_normal_initializer())
# Or if the variable is only local and not trainable
# w = tf.Variable(initial_value=tf.random_normal(5,), dtype=tf.float32, trainable=False)
return 2 * (x * w)
x1 = tf.placeholder(shape=(), dtype=tf.float32, name='X1')
x2 = tf.placeholder(shape=(), dtype=tf.float32, name='X2')
some_function_tpl = tf.make_template('some_function', some_function)
y1 = some_function_tpl(x1)
y2 = some_function_tpl(x2)
init = tf.global_variables_initializer()
with tf.Session() as sess:
sess.run(init)
print(*sess.run([y1, y2], feed_dict=x1: 1, x2: 10), sep='n')
Nice! Thanks a lot. Now the only thing that's unclear is how you do this when the "subgraph" already has values associated with the variables (eg. you restored the state withnew_saver = tf.train.import_meta_graph(...)
andnew_saver.restore(sess, ...)
because it seems you can't do this with an active session..
– Peter
Nov 8 at 15:38
@Peter Yes, with Graph Editor you would have to save and close the session before editing and restore it back after. But the variable object is really the same, so everything should work fine if you do that.
– jdehesa
Nov 8 at 15:54
add a comment |
up vote
1
down vote
accepted
There is the Graph Editor module to help with this sort of operations. Its main disadvantage is that you cannot have a running session while you modify the graph. However, you can checkpoint the session, modify the graph and the restore it back if you need so.
The problem with what you want is that you basically need to replicate a subgraph except you do no want to replicate variables. So you can simply exclude variable types (mainly Variable
, VariableV2
and maybe VarHandleOp
, although I threw in a few more I found in TensorFlow code). You can do that with a function like this:
import tensorflow as tf
# Receives the outputs to recalculate and the input replacements
def replicate_subgraph(outputs, mappings):
# Types of operation that should not be replicated
# Taken from tensorflow/python/training/device_setter.py
NON_REPLICABLE = 'Variable', 'VariableV2', 'AutoReloadVariable',
'MutableHashTable', 'MutableHashTableV2',
'MutableHashTableOfTensors', 'MutableHashTableOfTensorsV2',
'MutableDenseHashTable', 'MutableDenseHashTableV2',
'VarHandleOp', 'BoostedTreesEnsembleResourceHandleOp'
# Find subgraph ops
ops = tf.contrib.graph_editor.get_backward_walk_ops(outputs, stop_at_ts=mappings.keys())
# Exclude non-replicable operations
ops_replicate = [op for op in ops if op.type not in NON_REPLICABLE]
# Make subgraph viewitems
sgv = tf.contrib.graph_editor.make_view(*ops_replicate)
# Make the copy
_, info = tf.contrib.graph_editor.copy_with_input_replacements(sgv, mappings)
# Return new outputs
return info.transformed(outputs)
For an example similar to yours (I edited it a bit so it is easy to see that the output is correct because the second value is ten times the first one).
import tensorflow as tf
def some_function(x):
w = tf.Variable(initial_value=tf.random_normal((5,)), dtype=tf.float32)
return 2 * (x * w)
x1 = tf.placeholder(shape=(), dtype=tf.float32, name='X1')
x2 = tf.placeholder(shape=(), dtype=tf.float32, name='X2')
y1 = some_function(x1)
y2, = replicate_subgraph([y1], x1: x2)
init = tf.global_variables_initializer()
with tf.Session() as sess:
sess.run(init)
print(*sess.run([y1, y2], feed_dict=x1: 1, x2: 10), sep='n')
Output:
[ 2.3356955 2.277849 0.58513653 2.0919807 -0.15102367]
[23.356955 22.77849 5.851365 20.919807 -1.5102367]
EDIT:
Here is another solution using tf.make_template
. This requires you to actually have the code for the function, but it is a cleaner and "more official" way of supporting subgraph reuse.
import tensorflow as tf
def some_function(x):
w = tf.get_variable('W', (5,), initializer=tf.random_normal_initializer())
# Or if the variable is only local and not trainable
# w = tf.Variable(initial_value=tf.random_normal(5,), dtype=tf.float32, trainable=False)
return 2 * (x * w)
x1 = tf.placeholder(shape=(), dtype=tf.float32, name='X1')
x2 = tf.placeholder(shape=(), dtype=tf.float32, name='X2')
some_function_tpl = tf.make_template('some_function', some_function)
y1 = some_function_tpl(x1)
y2 = some_function_tpl(x2)
init = tf.global_variables_initializer()
with tf.Session() as sess:
sess.run(init)
print(*sess.run([y1, y2], feed_dict=x1: 1, x2: 10), sep='n')
Nice! Thanks a lot. Now the only thing that's unclear is how you do this when the "subgraph" already has values associated with the variables (eg. you restored the state withnew_saver = tf.train.import_meta_graph(...)
andnew_saver.restore(sess, ...)
because it seems you can't do this with an active session..
– Peter
Nov 8 at 15:38
@Peter Yes, with Graph Editor you would have to save and close the session before editing and restore it back after. But the variable object is really the same, so everything should work fine if you do that.
– jdehesa
Nov 8 at 15:54
add a comment |
up vote
1
down vote
accepted
up vote
1
down vote
accepted
There is the Graph Editor module to help with this sort of operations. Its main disadvantage is that you cannot have a running session while you modify the graph. However, you can checkpoint the session, modify the graph and the restore it back if you need so.
The problem with what you want is that you basically need to replicate a subgraph except you do no want to replicate variables. So you can simply exclude variable types (mainly Variable
, VariableV2
and maybe VarHandleOp
, although I threw in a few more I found in TensorFlow code). You can do that with a function like this:
import tensorflow as tf
# Receives the outputs to recalculate and the input replacements
def replicate_subgraph(outputs, mappings):
# Types of operation that should not be replicated
# Taken from tensorflow/python/training/device_setter.py
NON_REPLICABLE = 'Variable', 'VariableV2', 'AutoReloadVariable',
'MutableHashTable', 'MutableHashTableV2',
'MutableHashTableOfTensors', 'MutableHashTableOfTensorsV2',
'MutableDenseHashTable', 'MutableDenseHashTableV2',
'VarHandleOp', 'BoostedTreesEnsembleResourceHandleOp'
# Find subgraph ops
ops = tf.contrib.graph_editor.get_backward_walk_ops(outputs, stop_at_ts=mappings.keys())
# Exclude non-replicable operations
ops_replicate = [op for op in ops if op.type not in NON_REPLICABLE]
# Make subgraph viewitems
sgv = tf.contrib.graph_editor.make_view(*ops_replicate)
# Make the copy
_, info = tf.contrib.graph_editor.copy_with_input_replacements(sgv, mappings)
# Return new outputs
return info.transformed(outputs)
For an example similar to yours (I edited it a bit so it is easy to see that the output is correct because the second value is ten times the first one).
import tensorflow as tf
def some_function(x):
w = tf.Variable(initial_value=tf.random_normal((5,)), dtype=tf.float32)
return 2 * (x * w)
x1 = tf.placeholder(shape=(), dtype=tf.float32, name='X1')
x2 = tf.placeholder(shape=(), dtype=tf.float32, name='X2')
y1 = some_function(x1)
y2, = replicate_subgraph([y1], x1: x2)
init = tf.global_variables_initializer()
with tf.Session() as sess:
sess.run(init)
print(*sess.run([y1, y2], feed_dict=x1: 1, x2: 10), sep='n')
Output:
[ 2.3356955 2.277849 0.58513653 2.0919807 -0.15102367]
[23.356955 22.77849 5.851365 20.919807 -1.5102367]
EDIT:
Here is another solution using tf.make_template
. This requires you to actually have the code for the function, but it is a cleaner and "more official" way of supporting subgraph reuse.
import tensorflow as tf
def some_function(x):
w = tf.get_variable('W', (5,), initializer=tf.random_normal_initializer())
# Or if the variable is only local and not trainable
# w = tf.Variable(initial_value=tf.random_normal(5,), dtype=tf.float32, trainable=False)
return 2 * (x * w)
x1 = tf.placeholder(shape=(), dtype=tf.float32, name='X1')
x2 = tf.placeholder(shape=(), dtype=tf.float32, name='X2')
some_function_tpl = tf.make_template('some_function', some_function)
y1 = some_function_tpl(x1)
y2 = some_function_tpl(x2)
init = tf.global_variables_initializer()
with tf.Session() as sess:
sess.run(init)
print(*sess.run([y1, y2], feed_dict=x1: 1, x2: 10), sep='n')
There is the Graph Editor module to help with this sort of operations. Its main disadvantage is that you cannot have a running session while you modify the graph. However, you can checkpoint the session, modify the graph and the restore it back if you need so.
The problem with what you want is that you basically need to replicate a subgraph except you do no want to replicate variables. So you can simply exclude variable types (mainly Variable
, VariableV2
and maybe VarHandleOp
, although I threw in a few more I found in TensorFlow code). You can do that with a function like this:
import tensorflow as tf
# Receives the outputs to recalculate and the input replacements
def replicate_subgraph(outputs, mappings):
# Types of operation that should not be replicated
# Taken from tensorflow/python/training/device_setter.py
NON_REPLICABLE = 'Variable', 'VariableV2', 'AutoReloadVariable',
'MutableHashTable', 'MutableHashTableV2',
'MutableHashTableOfTensors', 'MutableHashTableOfTensorsV2',
'MutableDenseHashTable', 'MutableDenseHashTableV2',
'VarHandleOp', 'BoostedTreesEnsembleResourceHandleOp'
# Find subgraph ops
ops = tf.contrib.graph_editor.get_backward_walk_ops(outputs, stop_at_ts=mappings.keys())
# Exclude non-replicable operations
ops_replicate = [op for op in ops if op.type not in NON_REPLICABLE]
# Make subgraph viewitems
sgv = tf.contrib.graph_editor.make_view(*ops_replicate)
# Make the copy
_, info = tf.contrib.graph_editor.copy_with_input_replacements(sgv, mappings)
# Return new outputs
return info.transformed(outputs)
For an example similar to yours (I edited it a bit so it is easy to see that the output is correct because the second value is ten times the first one).
import tensorflow as tf
def some_function(x):
w = tf.Variable(initial_value=tf.random_normal((5,)), dtype=tf.float32)
return 2 * (x * w)
x1 = tf.placeholder(shape=(), dtype=tf.float32, name='X1')
x2 = tf.placeholder(shape=(), dtype=tf.float32, name='X2')
y1 = some_function(x1)
y2, = replicate_subgraph([y1], x1: x2)
init = tf.global_variables_initializer()
with tf.Session() as sess:
sess.run(init)
print(*sess.run([y1, y2], feed_dict=x1: 1, x2: 10), sep='n')
Output:
[ 2.3356955 2.277849 0.58513653 2.0919807 -0.15102367]
[23.356955 22.77849 5.851365 20.919807 -1.5102367]
EDIT:
Here is another solution using tf.make_template
. This requires you to actually have the code for the function, but it is a cleaner and "more official" way of supporting subgraph reuse.
import tensorflow as tf
def some_function(x):
w = tf.get_variable('W', (5,), initializer=tf.random_normal_initializer())
# Or if the variable is only local and not trainable
# w = tf.Variable(initial_value=tf.random_normal(5,), dtype=tf.float32, trainable=False)
return 2 * (x * w)
x1 = tf.placeholder(shape=(), dtype=tf.float32, name='X1')
x2 = tf.placeholder(shape=(), dtype=tf.float32, name='X2')
some_function_tpl = tf.make_template('some_function', some_function)
y1 = some_function_tpl(x1)
y2 = some_function_tpl(x2)
init = tf.global_variables_initializer()
with tf.Session() as sess:
sess.run(init)
print(*sess.run([y1, y2], feed_dict=x1: 1, x2: 10), sep='n')
edited Nov 8 at 15:22
answered Nov 8 at 15:07
jdehesa
20.7k33050
20.7k33050
Nice! Thanks a lot. Now the only thing that's unclear is how you do this when the "subgraph" already has values associated with the variables (eg. you restored the state withnew_saver = tf.train.import_meta_graph(...)
andnew_saver.restore(sess, ...)
because it seems you can't do this with an active session..
– Peter
Nov 8 at 15:38
@Peter Yes, with Graph Editor you would have to save and close the session before editing and restore it back after. But the variable object is really the same, so everything should work fine if you do that.
– jdehesa
Nov 8 at 15:54
add a comment |
Nice! Thanks a lot. Now the only thing that's unclear is how you do this when the "subgraph" already has values associated with the variables (eg. you restored the state withnew_saver = tf.train.import_meta_graph(...)
andnew_saver.restore(sess, ...)
because it seems you can't do this with an active session..
– Peter
Nov 8 at 15:38
@Peter Yes, with Graph Editor you would have to save and close the session before editing and restore it back after. But the variable object is really the same, so everything should work fine if you do that.
– jdehesa
Nov 8 at 15:54
Nice! Thanks a lot. Now the only thing that's unclear is how you do this when the "subgraph" already has values associated with the variables (eg. you restored the state with
new_saver = tf.train.import_meta_graph(...)
and new_saver.restore(sess, ...)
because it seems you can't do this with an active session..– Peter
Nov 8 at 15:38
Nice! Thanks a lot. Now the only thing that's unclear is how you do this when the "subgraph" already has values associated with the variables (eg. you restored the state with
new_saver = tf.train.import_meta_graph(...)
and new_saver.restore(sess, ...)
because it seems you can't do this with an active session..– Peter
Nov 8 at 15:38
@Peter Yes, with Graph Editor you would have to save and close the session before editing and restore it back after. But the variable object is really the same, so everything should work fine if you do that.
– jdehesa
Nov 8 at 15:54
@Peter Yes, with Graph Editor you would have to save and close the session before editing and restore it back after. But the variable object is really the same, so everything should work fine if you do that.
– jdehesa
Nov 8 at 15:54
add a comment |
Sign up or log in
StackExchange.ready(function ()
StackExchange.helpers.onClickDraftSave('#login-link');
);
Sign up using Google
Sign up using Facebook
Sign up using Email and Password
Post as a guest
StackExchange.ready(
function ()
StackExchange.openid.initPostLogin('.new-post-login', 'https%3a%2f%2fstackoverflow.com%2fquestions%2f53209495%2ftensorflow-copy-existing-graph-into-new-graph-multiple-times%23new-answer', 'question_page');
);
Post as a guest
Sign up or log in
StackExchange.ready(function ()
StackExchange.helpers.onClickDraftSave('#login-link');
);
Sign up using Google
Sign up using Facebook
Sign up using Email and Password
Post as a guest
Sign up or log in
StackExchange.ready(function ()
StackExchange.helpers.onClickDraftSave('#login-link');
);
Sign up using Google
Sign up using Facebook
Sign up using Email and Password
Post as a guest
Sign up or log in
StackExchange.ready(function ()
StackExchange.helpers.onClickDraftSave('#login-link');
);
Sign up using Google
Sign up using Facebook
Sign up using Email and Password
Sign up using Google
Sign up using Facebook
Sign up using Email and Password
Post as a guest
pR0BfCrRuZDOWLrbmAo09tnmCZBXksJjmjcyDD
What is it that you start with exactly? A
GraphDef
? Or just some graph in memory from where you want to duplicate a subgraph (delimited by an input and an output) and connect it somewhere else? Also do you need to do this while you are creating the graph or within an alive session?– jdehesa
Nov 8 at 14:23
I start with access to tensors
x
,y
, and nothing else (edited question to clarify, thanks)– Peter
Nov 8 at 14:25